Lanelet2 for nuScenes: Enabling Spatial Semantic Relationships and Diverse Map-based Anchor Paths
by Alexander Naumann*, Felix Hertlein*, Daniel Grimm, Maximilian Zipfl, Steffen Thoma, Achim Rettinger, Lavdim Halilaj, Juergen Luettin, Stefan Schmid, Holger Caesar.Paper Code Venue Notes
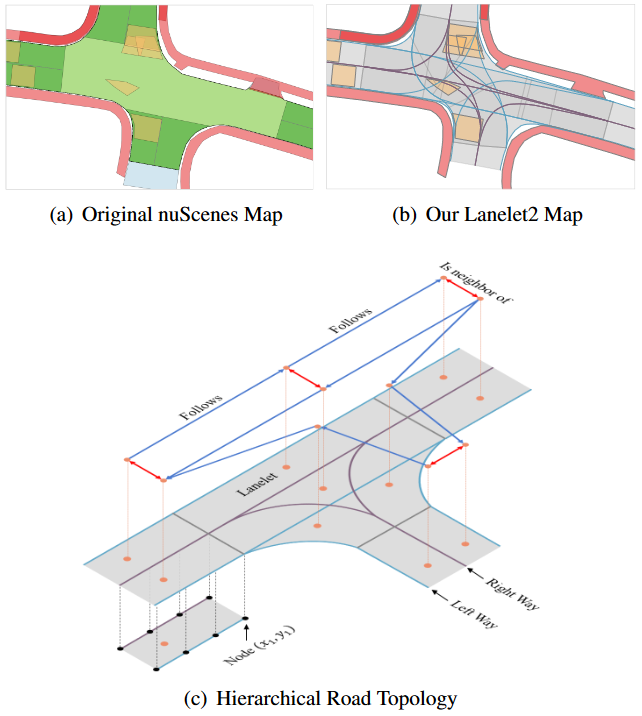
Abstract
Motion prediction and planning are key components to enable autonomous driving. Although high definition (HD) maps provide important contextual information that constrains the action space of traffic participants, most approaches are not able to fully exploit this heterogeneous information. In this work, we enrich the existing road geometry of the popular nuScenes dataset and convert it into the open-source map framework Lanelet2. This allows easy access to the road topology and thus, enables the usage of (1) spatial semantic information, such as agents driving on intersecting roads and (2) map-generated anchor paths for target vehicles that can help to improve trajectory prediction performance. Further, we present DMAP, a simple, yet effective approach for diverse map-based anchor path generation and filtering. We show that combining DMAP with ground truth velocity profile information yields high-quality motion prediction results on nuScenes (MinADE5=1.09, MissRate5,2=0.18, Offroad rate=0.00). While it is obviously unfair to compare us against the state-of-the-art, it shows that our HD map accurately depicts the road geometry and topology. Future approaches can leverage this by focusing on data-driven sampling of map-based anchor paths and estimating velocity profiles. Moreover, our HD map can be used for map construction tasks and supplement perception. Code and data are made publicly available at https://felixhertlein.github.io/lanelet4nuscenes.