Data Scaling Laws for End-to-End Autonomous Driving
by Alexander Naumann*, Xunjiang Gu*, Tolga Dimlioglu*, Mariusz Bojarski, Alperen Degirmenci, Alexander Popov, Devansh Bisla, Marco Pavone, Urs Müller, Boris Ivanovic.arXiv Venue Notes
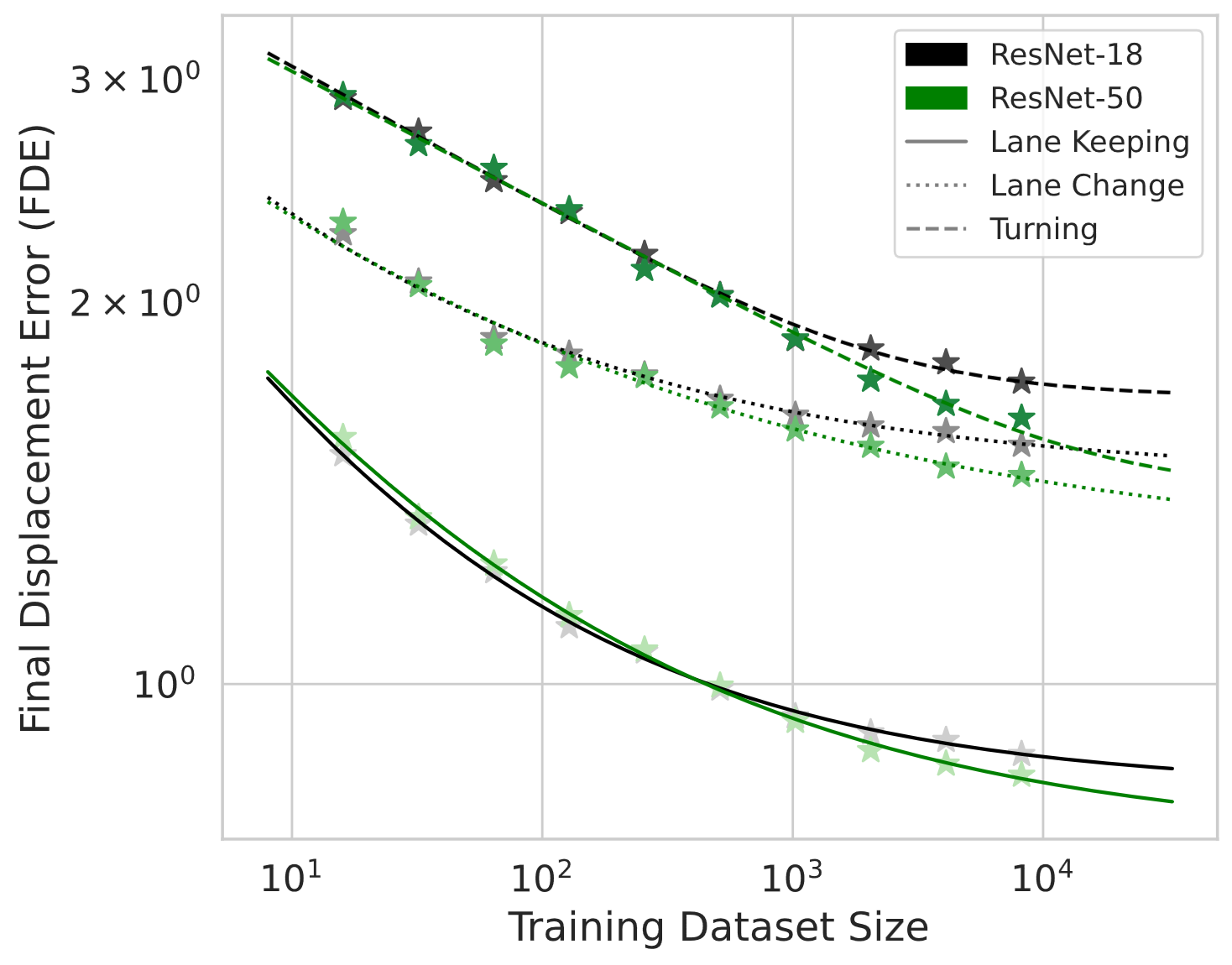
Abstract
Autonomous vehicle (AV) stacks have traditionally relied on decomposed approaches, with separate modules handling perception, prediction, and planning. However, this design introduces information loss during inter-module communication, increases computational overhead, and can lead to compounding errors. To address these challenges, recent works have proposed architectures that integrate all components into an end-to-end differentiable model, enabling holistic system optimization. This shift emphasizes data engineering over software integration, offering the potential to enhance system performance by simply scaling up training resources. In this work, we evaluate the performance of a simple end-to-end driving architecture on internal driving datasets ranging in size from 16 to 8192 hours with both open-loop metrics and closed-loop simulations. Specifically, we investigate how much additional training data is needed to achieve a target performance gain, e.g., a 5% improvement in motion prediction accuracy. By understanding the relationship between model performance and training dataset size, we aim to provide insights for data-driven decision-making in autonomous driving development.